Case Studies
Genialis works with pharma and biopharma, diagnostics companies, and clinical researchers to develop and commercialize RNA Biomarkers
Our expertise extends beyond our proprietary biomarker pipeline. We collaborate with partners to design and execute biomarker programs tailored for specific goals, including:
Enhancing translational decision-making
Optimizing clinical trial design
Developing new assays
Improving patient care
A biopharma company developing an innovative RAS inhibitor turned to Genialis for enhanced patient stratification beyond standard mutation-based approaches—and deeper insights into their drug’s mechanism of action
Approach
Pinpoint Pathways
We collaborated to identify critical biological pathways in solid tumor patientsRapidly Configure the Algorithm
Leveraging the Genialis™ Supermodel and Genialis™ krasID, we delivered a proof-of-concept biomarker algorithm tuned to the drug and indication—all in under six weeksRefine with Real-World, Validate on In-Patient Data
The algorithm was fine-tuned using human datasets and retrospectively validated with Phase 1 clinical resultsEnable Clinical Deployment
Our R&D team is now partnering with the company’s diagnostic division to implement the biomarker for clinical trial assay development
Result
Armed with a powerful tool to better understand their drug’s mechanism of action and predict patient response, the biopharma partner successfully integrated an advanced biomarker strategy into their clinical program—accelerating progress toward regulatory approval.
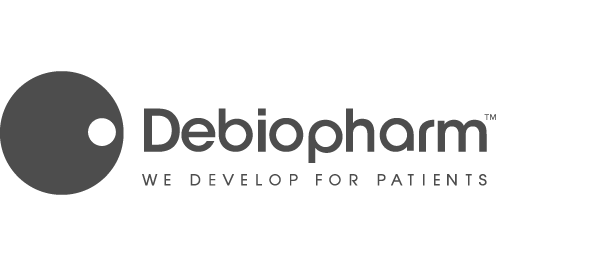
Debiopharm, a leader in DNA Damage Repair (DDR) therapeutics, is collaborating with Genialis to develop a model aimed at optimizing patient stratification for their DDR-targeting drugs. Historically, the complexity of DDR biology has limited the durability of monotherapies, necessitating patient selection and rational combinations, both requiring accurate and information-rich biomarkers.
Approach
Pinpoint Pathways
We dissected DDR’s complexities to identify critical biological processes and interactions influencing therapy response and resistanceRapidly Configure the Algorithm
We configured a biomarker algorithm comprising numerous biological modules representing various biologies contributing to DDR therapeutic vulnerabilityRefine and Validate on In-vivo Data
Developing a Debiopharm proprietary model using existing translational and clinical data to enhance the biomarker’s predictive power for patient responseExplore Clinical Deployment
Genialis continues to collaborate with Debiopharm to refine the biomarker and explore its potential integration into clinical trials
Result
In early 2025, Genialis announced an extension of its collaboration with Debiopharm to develop a predictive biomarker for WEE1-targeted therapy. Debiopharm’s WEE1 asset is currently in Phase 1 clinical research and is being studied as monotherapy and in multiple combinations in collaboration with international partners.
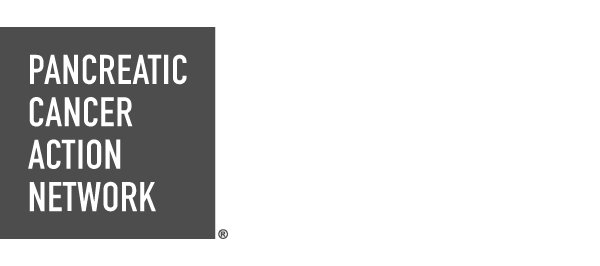
Genialis is proud to partner with the Pancreatic Cancer Action Network (PanCAN) to advance biomarker solutions for patients with pancreatic cancer. Through PanCAN’s “Know Your Tumor” program, we analyzed 493 pancreatic adenocarcinoma samples using Genialis krasID.
This large-scale analysis uncovered varying probabilities of response to KRAS inhibitors across multiple patient genotypes (including KRAS G12D and wild-type). These findings highlight how krasID could guide more personalized treatment.
Moving forward, we’re collaborating with PanCAN to refine krasID so clinicians can select the most effective therapies, ultimately improving patient outcomes and quality of life.
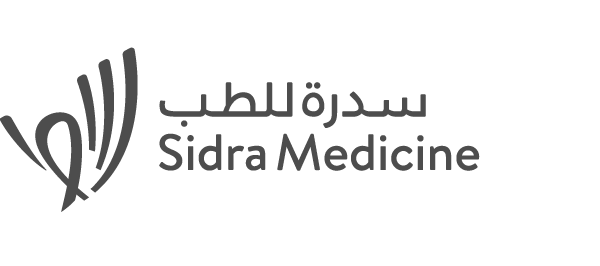
Genialis has teamed up with Sidra Medicine to validate biomarkers that predict how patients from the Middle East will respond to gastrointestinal cancer treatments.
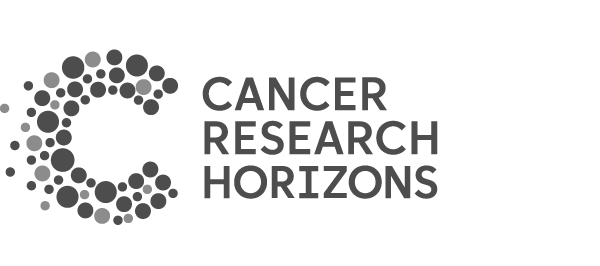
In 2023, Genialis partnered with Cancer Research Horizons (CRH), the innovation arm of Cancer Research UK. CRH provided anonymized, curated datasets with ‘omics and clinical metadata to support the training and validation of Genialis RNA biomarkers.
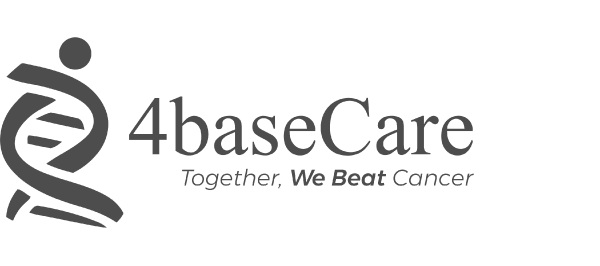
Genialis and 4baseCare are working together to develop biomarkers that predict patient responses to standard-of-care treatments in Asia and the Middle East.
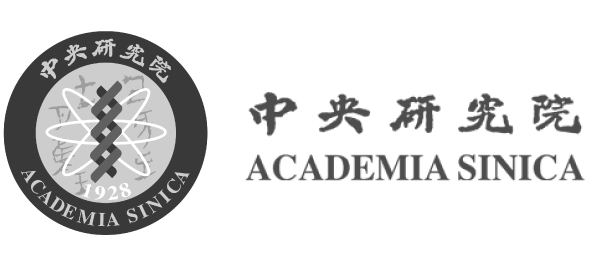
Genialis and Academia Sinica have launched an NSCLC program to identify the best treatments for early-stage and TKI-resistant patients in East Asia.