We transform transcriptomic data into actionable patient subgroups and treatment recommendations
We model fundamental biology using human data and machine learning to ensure successful development of new drugs, and inform targeted treatment decisions for better outcomes.
We are building the next generation of cancer biomarkers based on gene expression data. They incorporate dozens to hundreds of genes into machine learning classifiers capable of capturing therapeutically meaningful relationships between genes and pathways.
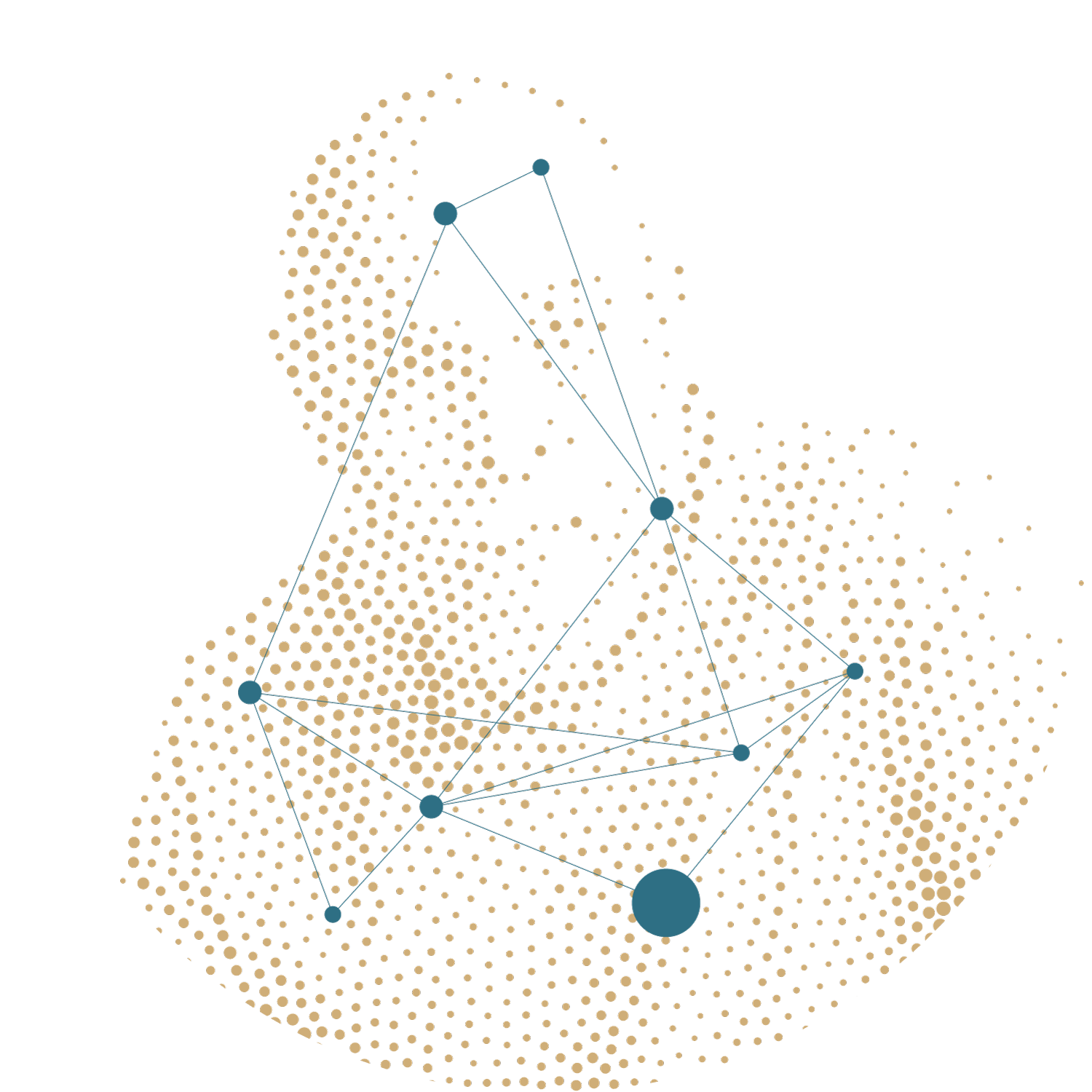
Answer questions like:
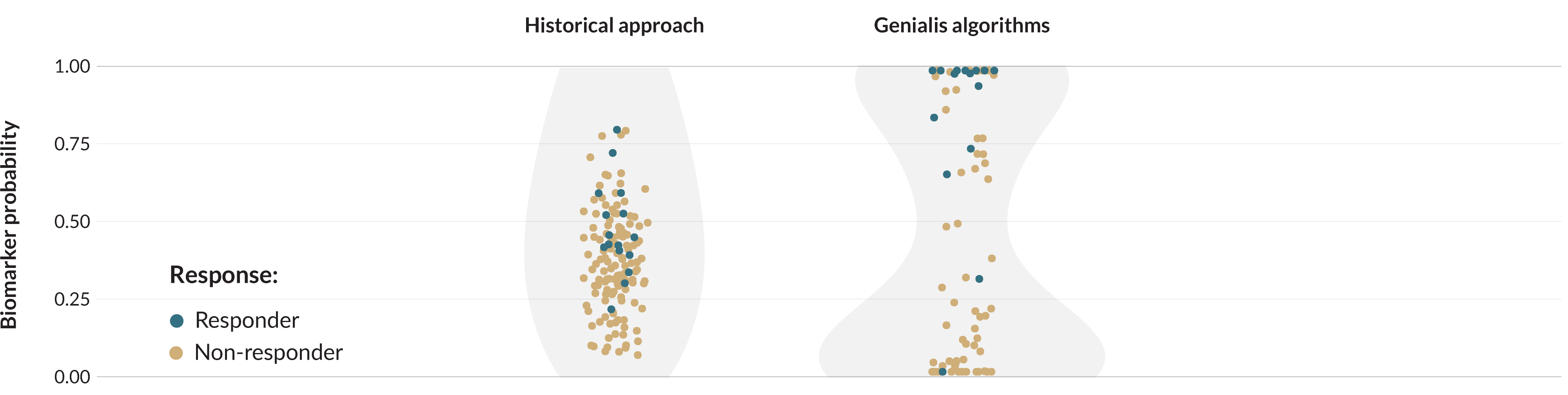
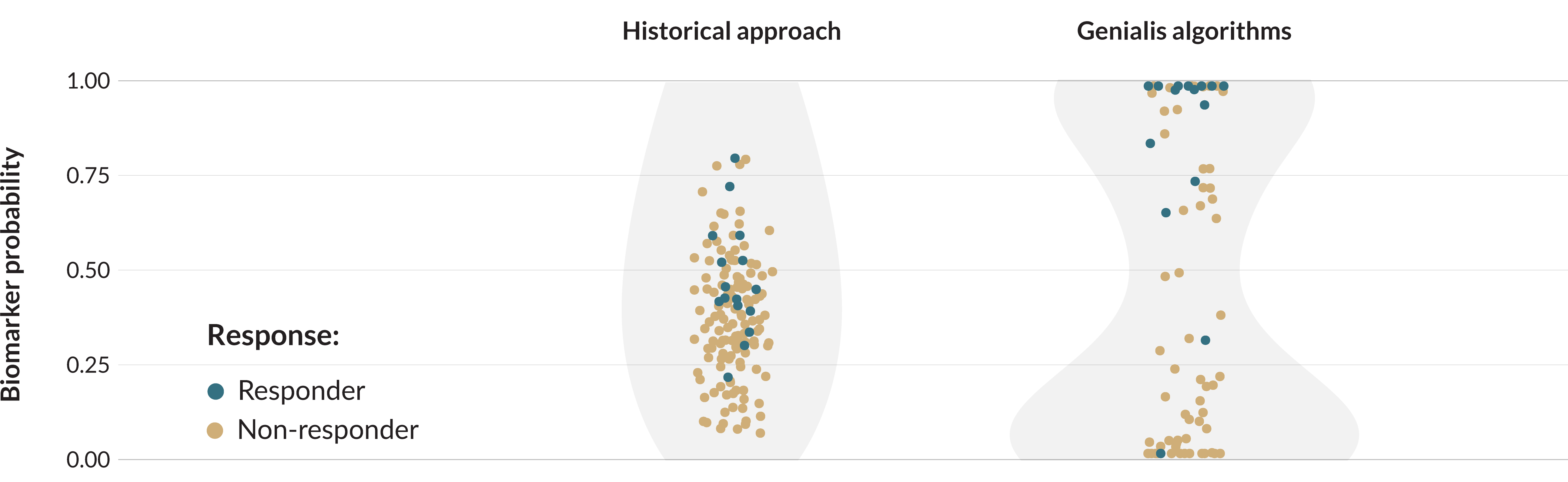
RNA Biomarkers:
The Future is Now
Why RNA?
Genialis focuses on RNA because gene expression-based biomarkers give us the best of both worlds: insight into disease drivers at the genome level and dynamic states defined by the transcriptome. The additional information on pathways and processes, especially compared with DNA or protein biomarkers, are especially relevant to predicting treatment outcomes of complex diseases.
Genialis prefers RNA biomarkers for several reasons
How biomarkers predict response to targeted treatment
![]() DNA biomarker |
![]() RNA biomarker |
|
Biology | Driver gene disruption, mutational burden |
Pathway disruption, dysregulation, activation, suppression, etc PLUS: Driver gene disruption, mutational burden |
Feature | One or just a few DNA variants | Quantitative signatures comprising the expression and variants of dozens of genes |
Algorithm | Binary mutation status | Machine learning classifier |
Capability | Necessary but insufficient: Describes the status of a drug target or disease driver, but does not describe the biological state (or phenotype) of the disease. ![]() |
Predictive and explanatory: Captures complex interactions to define the biological state and treatment susceptibility of the disease. ![]() |
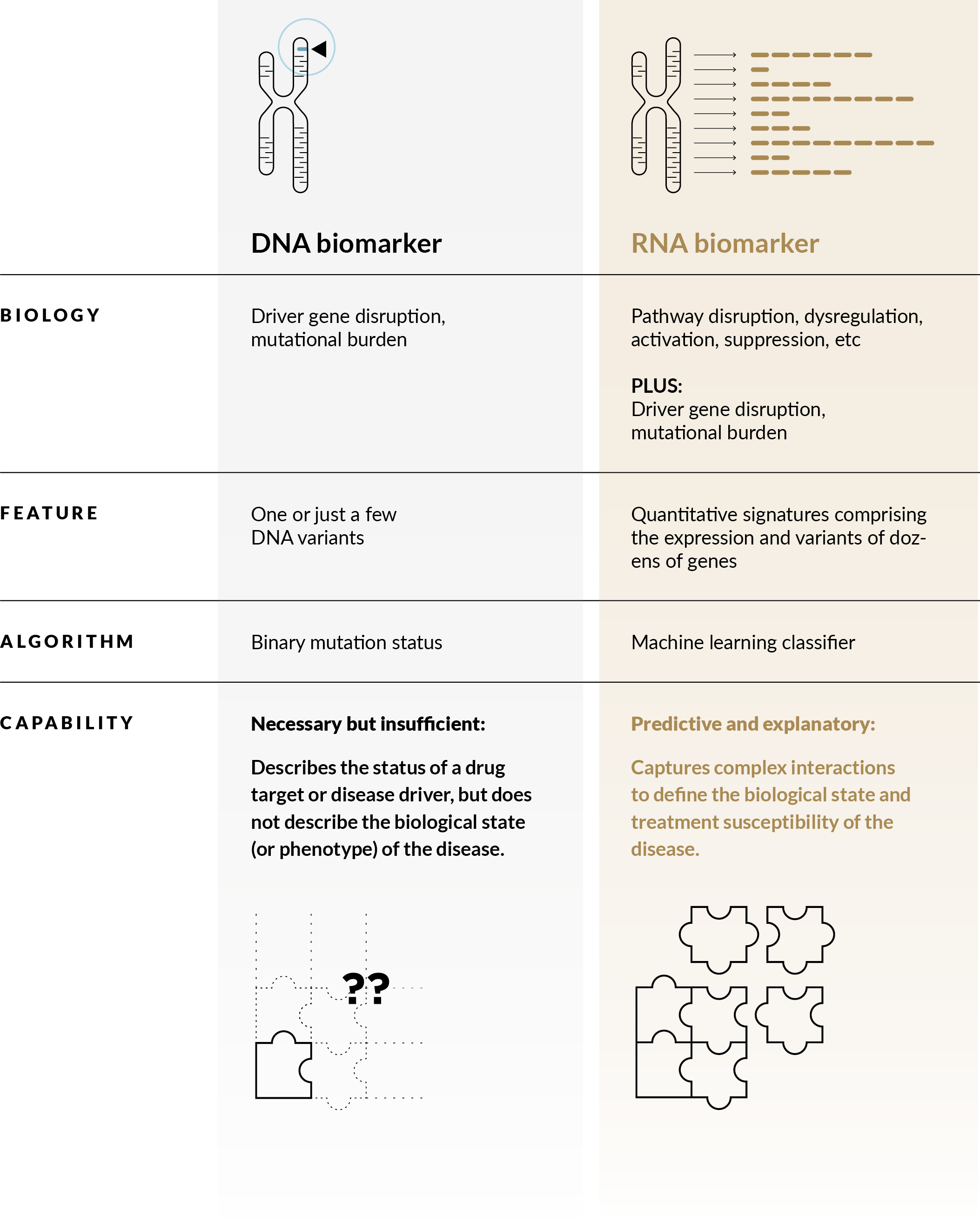