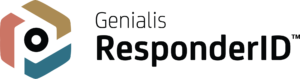
Genialis ResponderID™ is a framework for biomarker discovery by modeling complex disease biology. ResponderID marries a novel “people first” approach to biomarker conceptualization with proprietary technology, validated signatures, and human data. The result is biomarkers that work in real patient populations and scale across clinical settings.
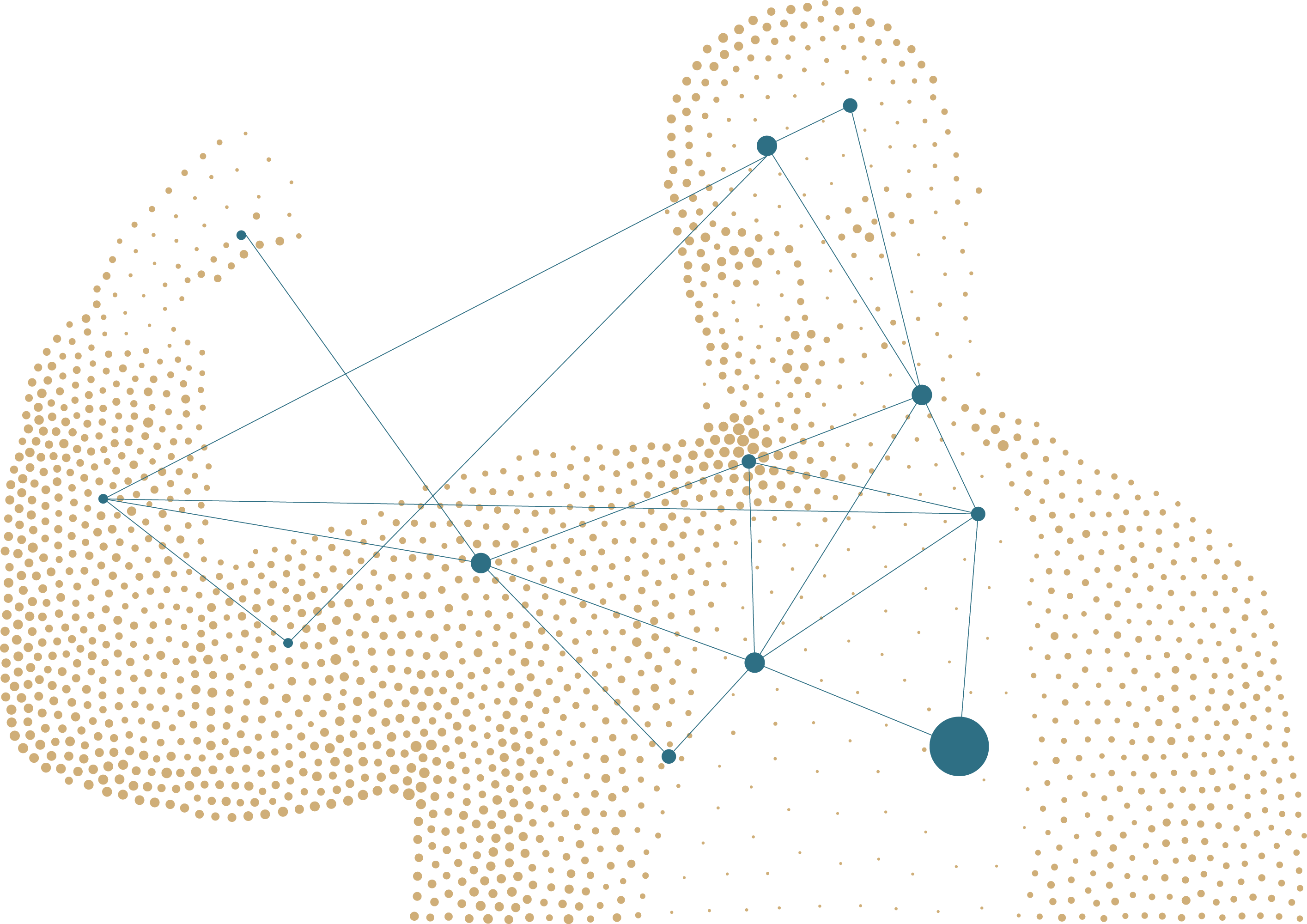
ResponderID includes algorithms that predict response to therapies targeting the tumor microenvironment, classify MSI, TMB, and other state-of-the-art immune signatures, and support the development of emerging drugs like KRAS inhibitors.
ResponderID is uniquely capable of developing biomarkers for drugs by modeling disease biology rather than relying on treatment response. Adhering to the FDA’s Good Machine Learning Practice guidelines and numerous international standards, our biomarkers are built to work for patients. These “just in time” biomarkers are especially well suited for helping new therapies reach the right patients who need them most.
Putting people first, Genialis provides a comprehensive picture of each patient’s disease. Genialis biomarkers not only stratify which patients are likely to respond to a particular drug but illuminate why a patient may or may not respond.
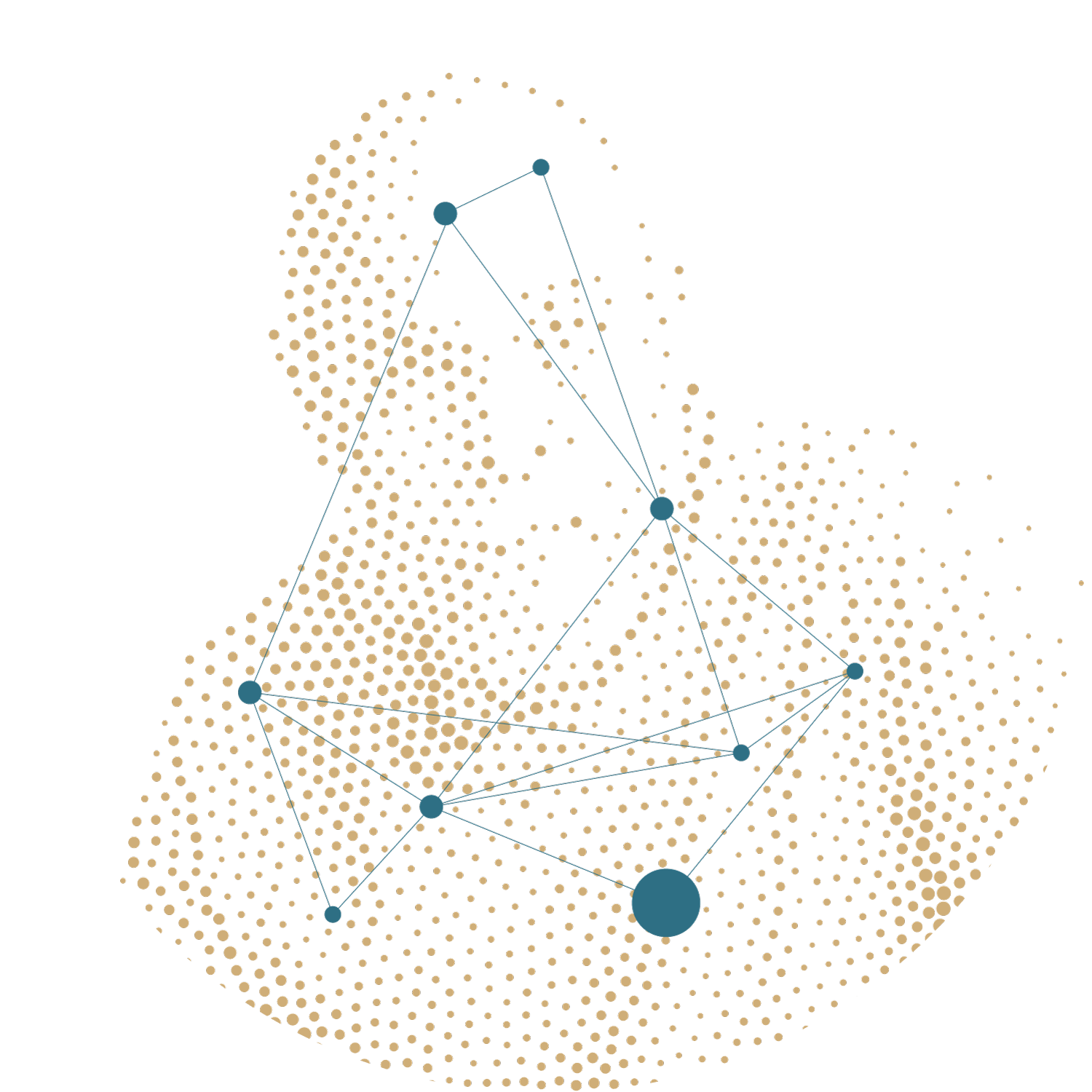
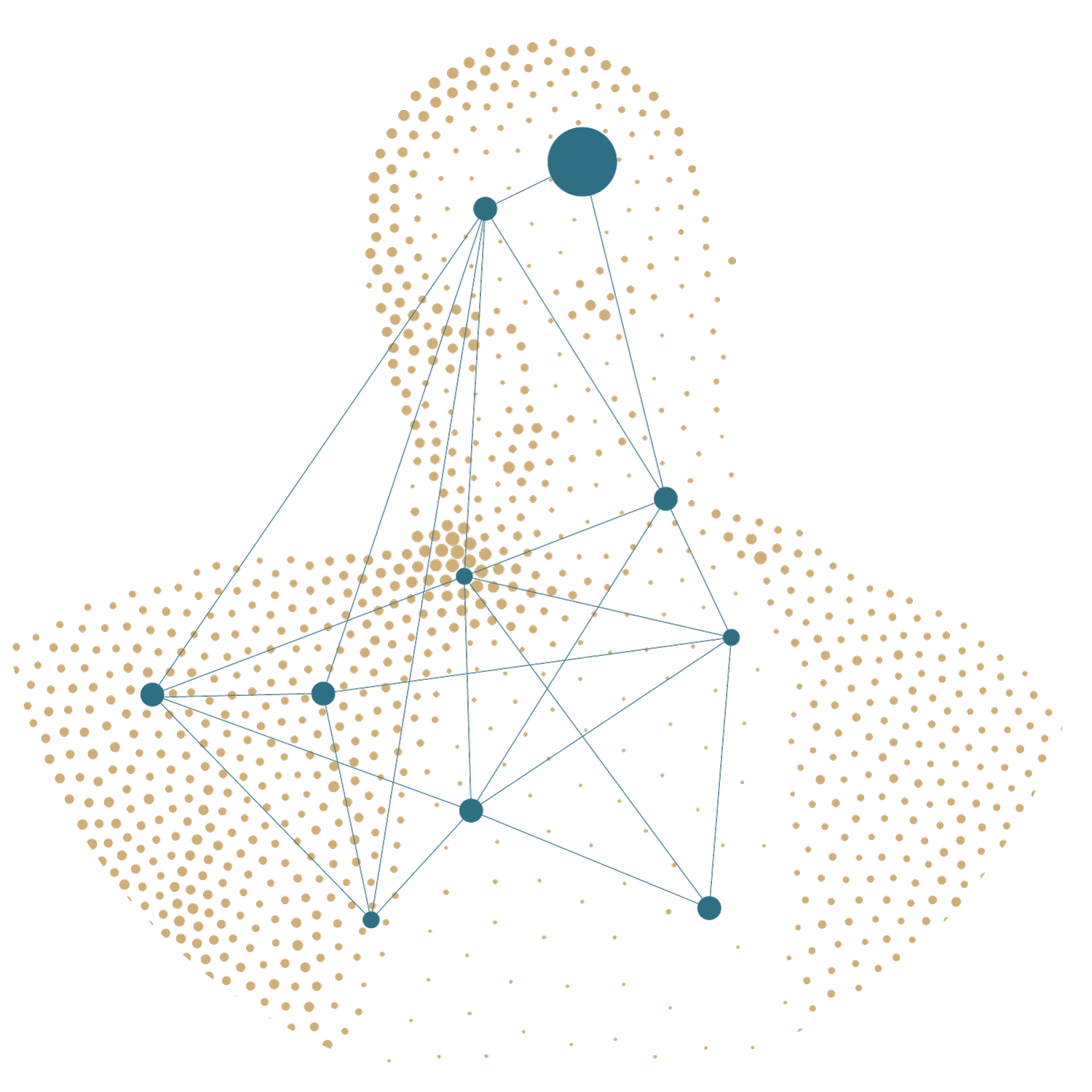
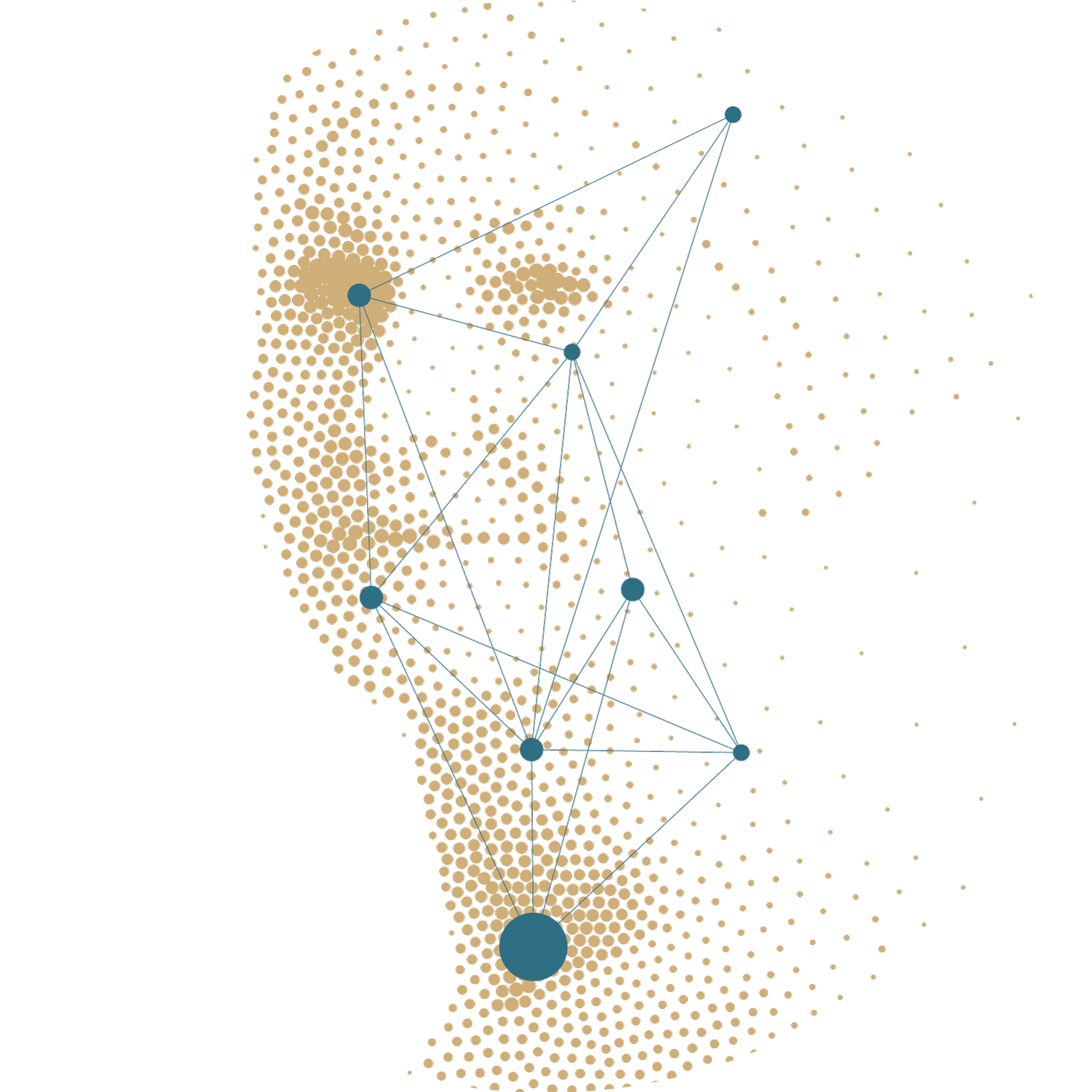
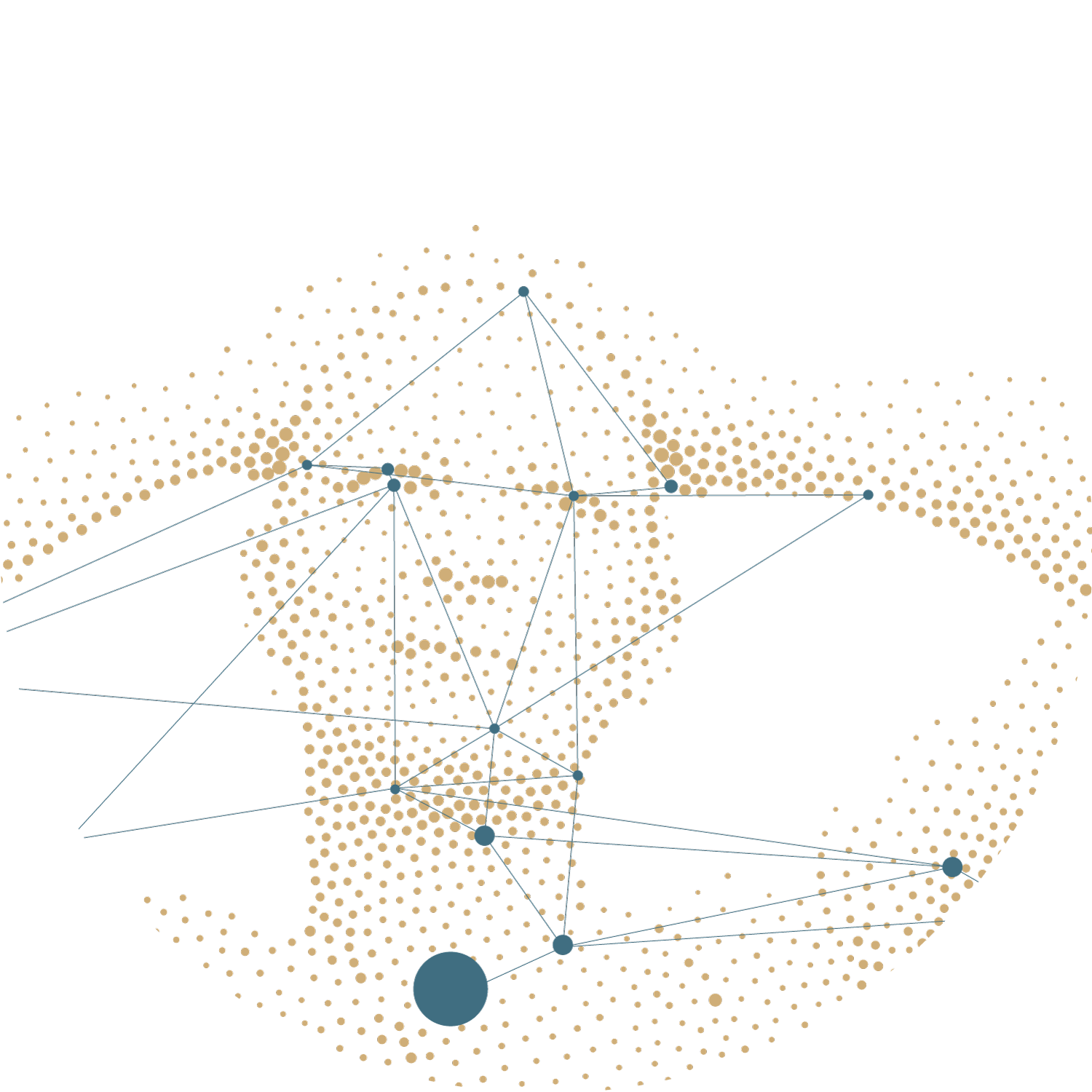
"We are so focused on getting the science right, because when we do, it changes lives."
CTO and Co-founder of Genialis
Key Advantages
Why is ResponderID better than other biomarker approaches?
- Start the development early, even before the first clinical trial results are collected.
- Solve the problem of small clinical datasets with other retrospective and proxy data.
- Create models that are useful for different types of drugs and different cancer indications.
- Hypotheses testing and data acquisition work together for rapid discovery and development.
- Performance is evaluated on retrospective, prospective, and proxy data
- Superb software engineering reliably clears regulatory hurdles
- RNA-based, thus extremely information rich
- Representative, with dozens to hundreds of genes to more fully capture complex biology
- Robust, such thatAI captures redundant and non-linear relationships between genes
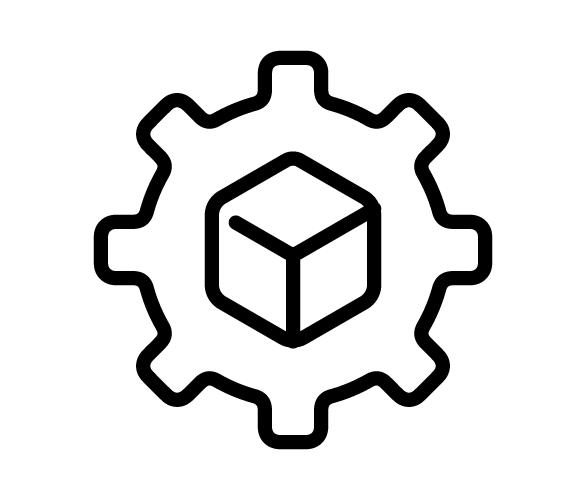
Proprietary technologies
bioinformatics software, machine learning sandbox
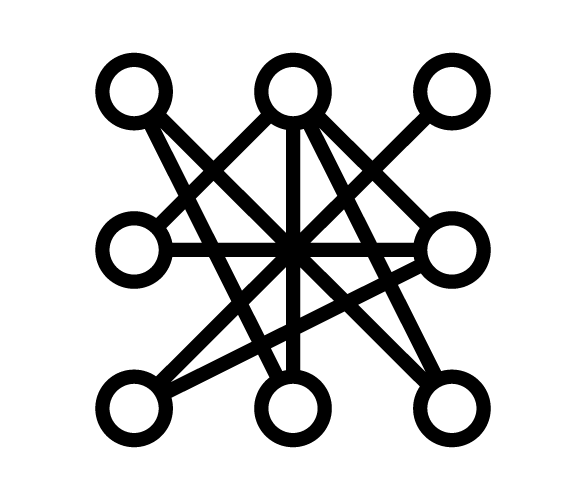
Validated algorithms
published signatures & standard of care biomarkers
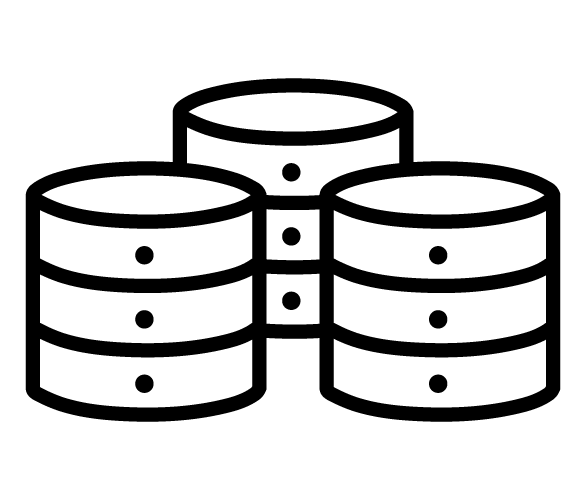
Harmonized data assets
machine learning-ready ‘omics & clinical metadata
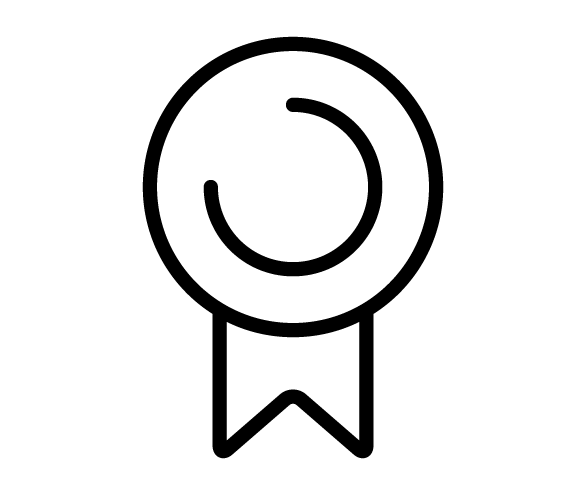
Expert services
data analytics, strategic planning, program management